Artificial intelligence is making it possible for Street Views to be
mined for insights about the economy, politics and human behavior — just as text mining has done for years.
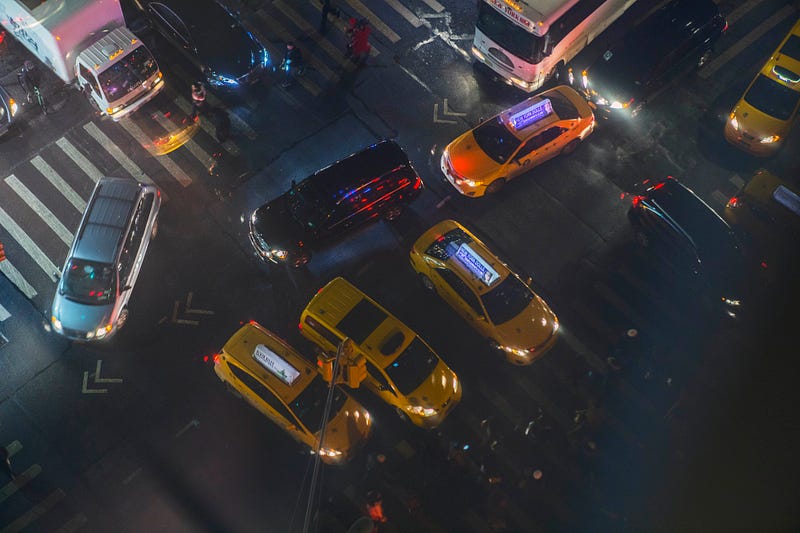
By Steve Lohr
What
vehicle is most strongly associated with Republican voting districts?
Extended-cab pickup trucks. For Democratic districts? Sedans.
Those
conclusions may not be particularly surprising. After all, market
researchers and political analysts have studied such things for decades.
But
what is surprising is how researchers working on an ambitious project
based at Stanford University reached those conclusions: by analyzing 50
million images and location data from Google Street View, the
street-scene feature of the online giant’s mapping service.
For
the first time, helped by recent advances in artificial intelligence,
researchers are able to analyze large quantities of images, pulling out
data that can be sorted and mined to predict things like income,
political leanings and buying habits. In the Stanford study, computers
collected details about cars in the millions of images it processed,
including makes and models.
“All
of a sudden we can do the same kind of analysis on images that we have
been able to do on text,” said Erez Lieberman Aiden, a computer
scientist who heads a genomic research center at the Baylor School of
Medicine. He provided advice on one aspect of the Stanford project.
For
computers, as for humans, reading and observation are two distinct ways
to understand the world, Lieberman Aiden said. In that sense, he said,
“computers don’t have one hand tied behind their backs anymore.”
Text
has been easier for AI to handle, because words have discrete
characters — 26 letters, in the case of English. That makes it much
closer to the natural language of computers than the freehand chaos of
imagery. But image recognition technology, much of it developed by major
technology companies, has improved greatly in recent years.
The
Stanford project gives a glimpse at the potential. By pulling the
vehicles’ makes, models and years from the images, and then linking that
information with other data sources, the project was able to predict
factors like pollution and voting patterns at the neighborhood level.
“This
kind of social analysis using image data is a new tool to draw
insights,” said Timnit Gebru, who led the Stanford research effort. The
research has been published in stages, the most recent in late November
in the Proceedings of the National Academy of Sciences.
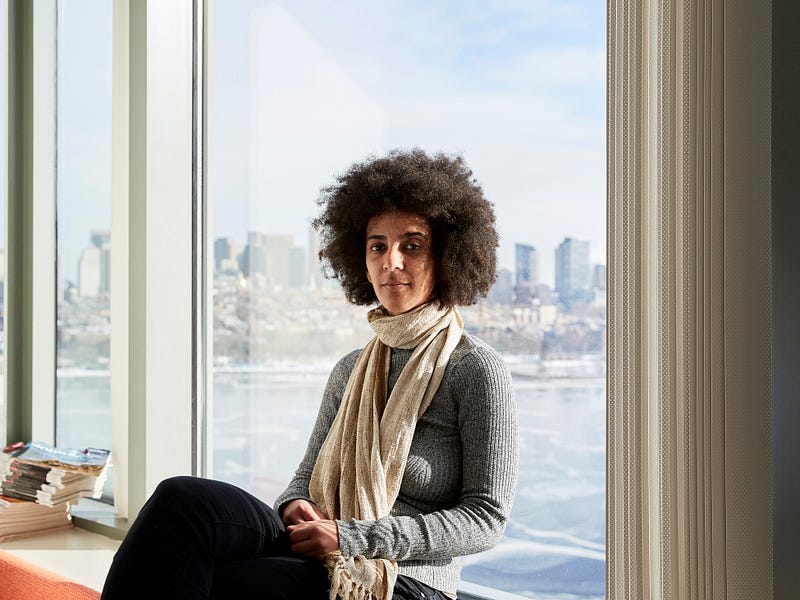
In
the end, the car-image project involved 50 million images of street
scenes gathered from Google Street View. In them, 22 million cars were
identified, and then classified into more than 2,600 categories like
their make and model, located in more than 3,000 ZIP codes and 39,000
voting districts.
But first, a database curated by humans had to train the AI software to understand the images.
The
researchers recruited hundreds of people to pick out and classify cars
in a sample of millions of pictures. Some of the online contractors did
simple tasks like identifying the cars in images. Others were car
experts who knew nuances like the subtle difference in the taillights on
the 2007 and 2008 Honda Accords.
“Collecting
and labeling a large data set is the most painful thing you can do in
our field,” said Gebru, who received her Ph.D. from Stanford in
September and now works for Microsoft Research.
But
without experiencing that data-wrangling work, she added, “you don’t
understand what is impeding progress in AI in the real world.”
Once
the car-image engine was built, its speed and predictive accuracy was
impressive. It successfully classified the cars in the 50 million images
in two weeks. That task would take a human expert, spending 10 seconds
per image, more than 15 years.
Identifying
so many car images in such detail was a technical feat. But it was
linking that new data set to public collections of socioeconomic and
environmental information, and then tweaking the software to spot
patterns and correlations, that makes the Stanford project part of what
computer scientists see as the broader application of image data.
“There
has been an explosion of computer vision research, but so far the
societal impact has been largely absent,” said Serge Belongie, a
computer scientist at Cornell Tech. “Being able to identify what is in a
photo is not science that advances our understanding of the world.”
The
Stanford car project generated a host of intriguing connections, not so
much startling revelations. In the most recent paper, and one published
earlier in the year by the Association for the Advancement of
Artificial Intelligence, these were among the predictive correlations:
—
The system was able to accurately predict income, race, education and
voting patterns at the ZIP code and precinct level in cities across the
country.
—
Car attributes (including mpg ratings) found that the greenest city in
America is Burlington, Vermont, while Casper, Wyoming, has the largest
per-capita carbon footprint.
—
Chicago is the city with the highest level of income segregation, with
large clusters of expensive and cheap cars in different neighborhoods;
Jacksonville, Florida, is the least segregated by income.
—
New York is the city with the most expensive cars. El Paso, Texas has
the highest percentage of Hummers. San Francisco has the highest
percentage of foreign cars.
Other
researchers have used Google Street View data for visual clues for
factors that influence urban development, ethnic shifts in local
communities and public health. But the Stanford project appears to have
used the most Street View images in the most detailed analysis so far.
The
significance of the project, experts say, is a proof of concept — that
new information can be gleaned from visual data with artificial
intelligence software and plenty of human help.
The
role of such research, they say, will be mainly to supplement
traditional information sources like the government’s American Community
Survey, the household surveys conducted by the Census Bureau.
This
kind of research, if it expands, will raise issues of data access and
privacy. The Stanford project only made predictions about neighborhoods,
not about individuals. But privacy concerns about Street View pictures
have been raised in Germany and elsewhere. Google says it handles
research requests for access to large amounts of its image data on a
case-by-case basis.
Onboard
cameras in cars are just beginning, as auto companies seek to develop
self-driving cars. Will some of the vast amounts of image data they
collect be available for research or kept proprietary?
Kenneth
Wachter, a professor of demography at the University of California,
Berkeley, said image-based studies could be a big help now that public
response rates to sample surveys are declining. An AI-assisted visual
census, he said, could fill in gaps in the current data, but also
provide more timely insights than the traditional census, conducted
every 10 years, on hot topics in public policy like “the geography and
evolution of disadvantage and opportunity.”
To
Nikhil Naik, a computer scientist and research fellow at Harvard, who
has used Street View images in the study of urban environments, the
Stanford project points toward the future of image-fueled research.
“For
the first time in history, we have the technology to extract insights
from very large amounts of visual data,” Naik said. “But while the
technology is exciting, computer scientists need to work closely with
social scientists and others to make sure it’s useful.”
No comments:
Write comments