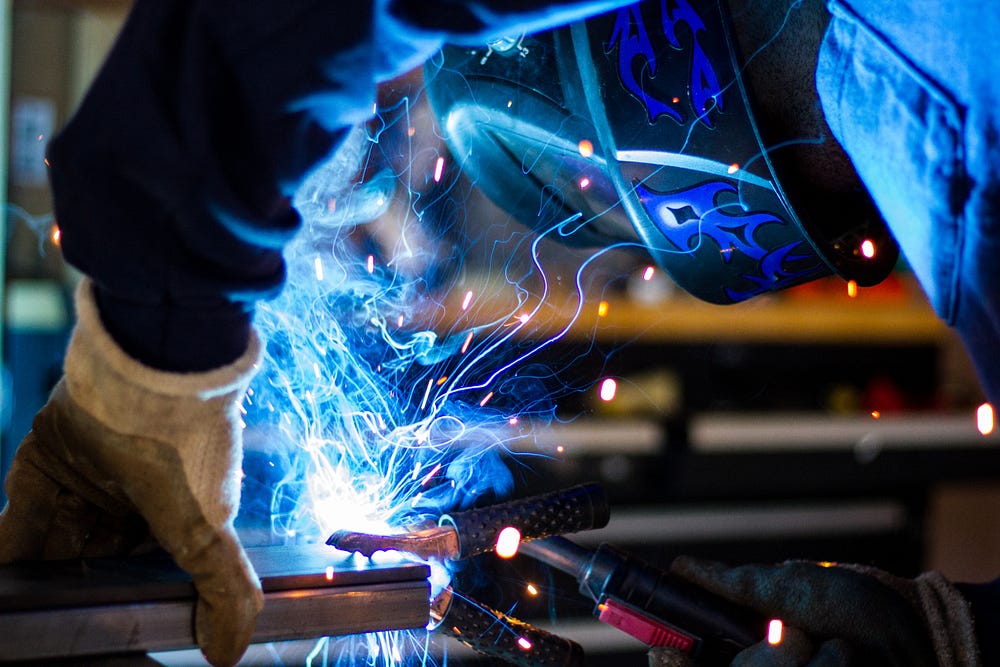
What It Takes to Train The Next Generation of Innovators
This article was published on GrowthX Academy’s Blog on August 28, 2017.
Sean Sheppard, founder of GrowthX Academy, discusses the critical skills for the upcoming “Innovation Economy”.
“How
do we educate people for a future we can’t predict?” It’s a question
that’s been on my mind a lot lately — and, it turns out, it’s been on Sean Sheppard‘s as well.
Sean is a serial entrepreneur, venture capitalist, and the founder of GrowthX
and the GrowthX Academy. He’s someone who’s been steeped in modern
sales, marketing and growth hacking methods, so I was excited to get the
chance to chat with him recently about the skills he believes will be
critical for the coming “Innovation Economy.”
The Problem: Our Outdated Education System
Most
of us have the sense that our education systems haven’t kept pace with
innovation. In our conversation, Sean explains how deeply behind we’ve
fallen:
“The modern education system was developed in the Age of Enlightenment to support the Industrial Revolution of the 19th century as a way to take people off of farms and educate them to work in factories. That’s why there are school bells. They’re meant to mimic factory whistles. That’s why we have the people lined up in desks, in rows, because that’s how an assembly line is constructed.”
As
Sean notes, this transition was critical. “In the 1900s, 40 percent of
the jobs in this country were farming jobs. Today, only 2 percent are
farming jobs.” Moving from an agriculture-driven society to an
industrial one required education systems that prepared students for the
kinds of jobs that were becoming available.
Sean
points out that we’re in a similar transition now. “Very soon, 40 to 50
percent of the jobs are going to be replaced by robots and automation.
We’re now entering what the World Economic Forum has called the fourth
industrial revolution: the ‘Innovation Economy.’”
The Four Factors of Future Effectiveness
So
what changes do we need to make to prepare for this coming transition?
What skills do students and professionals need to practice today to
build competency for future jobs? Sean highlights four pillars in
particular that form the basis of his approach at GrowthX (I’ll give you
a hint — none of them involve getting an MBA or liberal arts degree).
1. Mindset
I
was happy to hear Sean touch on mindset as one of his four pillars, as
it’s something I’ve been hammering into my team at Web Profits. Sean and
I agree — the future belongs to those who adopt a growth mindset,
rather than a fixed mindset.
None
of us can predict with 100% certainty what the future of the Innovation
Economy looks like (except maybe Mark Zuckerberg). Limiting yourself
with a fixed mindset — one that restricts you to considering things as
they are, not as they might be — could prevent you from identifying and
taking advantage of opportunities as they arise.
That’s
somewhat obvious, but Sean added an important note: “There is no
distinction between personal and professional development in the
Innovation Economy.” You can’t think of your future performance in terms
of your career alone. Embracing the growth mindset Sean suggests means
recognizing that every part of yourself — from your work to your health
and beyond — can, and should, be improved upon.
2. Mastery
Having
a growth-based mindset provides needed flexibility for an unclear
future. But mindset alone doesn’t fully answer the question of how you
prepare today for jobs that may not exist until tomorrow.
That’s
where competency-based education comes into play, according to Sean.
“Competency-based education models will be the future of education. It’s
the idea that we can measure people the same way you and I measure
marketing efforts in real-time. We can assess people quickly about
whether or not they’ve achieved the competency.”
Out
of competency, Sean suggests, mastery grows. “You acquire the
knowledge; there’s a framework for that. You practice it to demonstrate
that you can acquire the competencies, and then through the repetitive
iteration of that, you develop proficiency and then, ultimately,
mastery.”
Sean’s
model makes more sense when applied to a hypothetical job. Suppose you
want to become a growth hacker. There’s no “official” training program;
no university you can attend. So how do you prepare for this job?
According to Sean, you study the existing knowledge that’s available.
You identify and develop the core competencies involved in the job.
Then, through practice, iterative improvement and the simple investment
of time, you eventually achieve mastery.
The
beauty of this approach is that it’s available to everyone. Sean
states, “It’s about being a learn-it-all not a know-it-all. It’s about
understanding that the foundation of mastery is that you do not have to
be born with some natural level of inborn talent or set of skills.”
3. Career
Transforming
personal and professional mastery into a career will look different
than it used to, according to Sean. “As an individual you have to focus
on your career development, and as a manager and a leader, you have to
focus on helping people develop their careers.”
Long
tenures with a single company are practically nonexistent these days,
and our transition to the Innovation Economy will only accelerate this
change. Succeeding in this future — in whatever role you define
yourself — will require that you take an active role in managing your
career, as well as helping guide the careers of others.
4. Community
Mindset,
mastery and career are all factors you develop on your own. But, in
Sean’s opinion, where things really come together is in a focus on
community. “The modern education requires diversity of thought, opinion,
background, and experience from a whole host of different points of
view.”
Simply
put: you need a diverse community whose wisdom you can draw on to
advance your learning beyond what you’re capable of on your own.
Sean attempts to build communities like these through GrowthX (the
next session starts September 12th), but you can also cultivate your
own community by connecting with older mentors, those in other
industries and thought leaders you admire.
Now
isn’t the time to remain idle. By focusing on updating your mindset,
mastery, career and community, you’ll be ready to face whatever
challenges come your way in the new Innovation Economy.
This article was published on GrowthX Academy’s Blog on August 28, 2017.