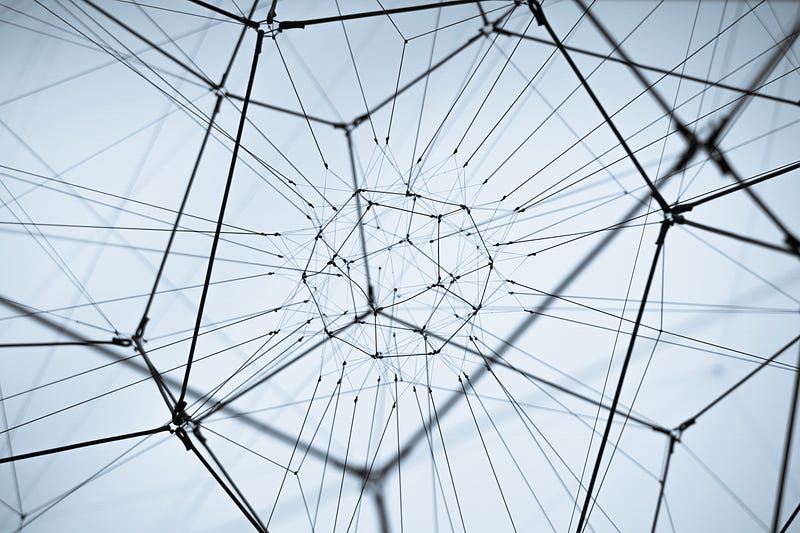
AI Innovation: Security and Privacy Challenges
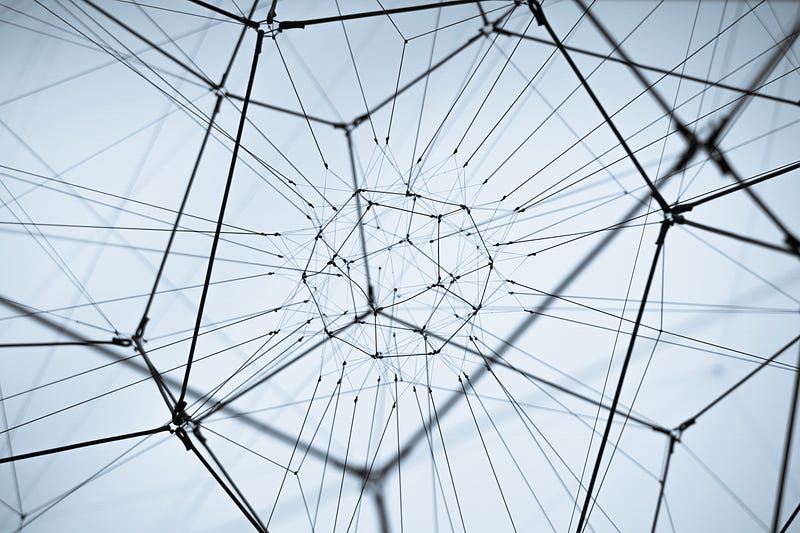
To
anyone working in technology (or, really, anyone on the Internet), the
term “AI” is everywhere. Artificial intelligence — technically, machine learning — is finding application in virtually every industry on the planet, from medicine and finance to entertainment and law enforcement.
As the Internet of Things (IoT) continues to expand, and the potential
for blockchain becomes more widely realized, ML growth will occur
through these areas as well.
While
current technical constraints limit these models from reaching “general
intelligence” capability, organizations continue to push the bounds of
ML’s domain-specific applications, such as image recognition and natural
language processing. Modern computing power (GPUs in particular) has contributed greatly to these recent developments — which is why it’s also worth noting that quantum computing will exponentialize this progress over the next several years.
Alongside
enormous growth in this space, however, has been increased criticism;
from conflating AI with machine learning to relying on those very
buzzwords to attract large investments, many “innovators” in this space have drawn criticism from technologists
as to the legitimacy of their contributions. Thankfully, there’s plenty
of room — and, by extension, overlooked profit — for innovation with
ML’s security and privacy challenges.
Reverse-Engineering
Machine learning models, much like any piece of software, are prone to theft and subsequent reverse-engineering. In late 2016, researchers
at Cornell Tech, the Swiss Institute EPFL, and the University of North
Carolina reverse-engineered a sophisticated Amazon AI by analyzing
its responses to only a few thousand queries; their clone replicated the
original model’s output with nearly perfect accuracy. The process is
not difficult to execute, and once completed, hackers will have
effectively “copied” the entire machine learning algorithm — which its
creators presumably spent generously to develop.
The risk this poses will only continue to grow. In addition
to the potentially massive financial costs of intellectual property
theft, this vulnerability also poses threats to national
security — especially as governments pour billions of dollars into autonomous weapon research.
While some researchers have suggested that increased model complexity is the best solution,
there hasn’t been nearly enough open work done in this space; it’s a
critical (albeit underpublicized) opportunity for innovation — all in
defense of the multi-billion-dollar AI sector.
Adversarial “Injection”
Machine
learning also faces the risk of adversarial “injection” — sending
malicious data that disrupts a neural network’s functionality. Last
year, for instance, researchers from four top universities confused
image recognition systems by adding small stickers onto a photo,
through what they termed Robust Physical Perturbation (RP2) attacks; the networks in question then misclassified the image. Another team at NYU showed a similar attack against a facial recognition system, which would allow a suspect individual to easily escape detection.
Not
only is this attack a threat to the network itself (i.e. consider this
against a self-driving car), but it’s also a threat to companies who
outsource their AI development and risk contractors putting their own
“backdoors” into the system. Jaime Blasco, Chief Scientist at security
company AlienVault, points out that this risk will only increase as the world depends more and more on machine learning. What would happen, for instance, if these flaws persisted in military systems? Law enforcement cameras? Surgical robots?
Training Data Privacy
Protecting the training data put into machine learning models is yet another area that needs innovation. Currently, hackers can reverse-engineer user data out of machine learning models
with relative ease. Since the bulk of a model’s training data is often
personally identifiable information —e.g. with medicine and
finance — this means anyone from an organized crime group to a business
competitor can reap economic reward from such attacks.
As machine learning models move to the cloud (i.e. self-driving cars), this becomes even more complicated; at
the same that users need to privately and securely send their data to
the central network, the network needs to make sure it can trust the
user’s data (so tokenizing the data via hashing, for instance, isn’t
necessarily an option). We can once again abstract this challenge with
everything from mobile phones to weapons systems.
Further,
as organizations seek personal data for ML research, their clients
might want to contribute to the work (e.g. improving cancer detection)
without compromising their privacy (e.g. providing an excess of PII that
just sits in a database). These two interests currently seem at
odds — but they also aren’t receiving much
focus, so we shouldn’t see this opposition as inherent. Smart redesign
could easily mitigate these problems.
Conclusion
In
short: it’s time some innovators in the AI space focused on its
security and privacy issues. With the world increasingly dependent on
these algorithms, there’s simply too much at stake — including a lot of
money for those who address these challenges.