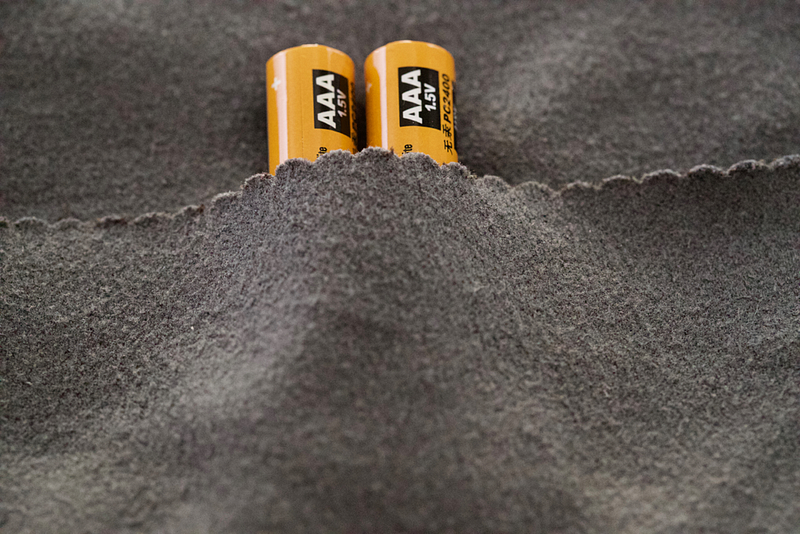
The Apple Battery Cover-Up: Triumph of Management, Failure of Leadership
This is a difficult post for me to write. It’s a post about Apple — yet it’s not the same...
We love our Work
This is a difficult post for me to write. It’s a post about Apple — yet it’s not the same...
In one year, HubSpot doubled the number of certified partners in its platform ecosystem and increased the number of apps...
In one of my earliest roles at a B2B startup, there were so many fires in a day, that if...
To anyone working in technology (or, really, anyone on the Internet), the term “AI” is everywhere. Artificial intelligence — technically,...
My name is Maxime Coutté. I’m 16 and I built my own VR headset with my best friends, Jonas Ceccon...
Face recognition is the latest trend when it comes to user authentication. Apple recently launched their new iPhone X which...
Everyday I commute to work or drive I notice the amount of drivers that still text and drive. Observing drivers...
This post was originally published on the RescueTime blog. Check us out for more like it. When exactly are we the...