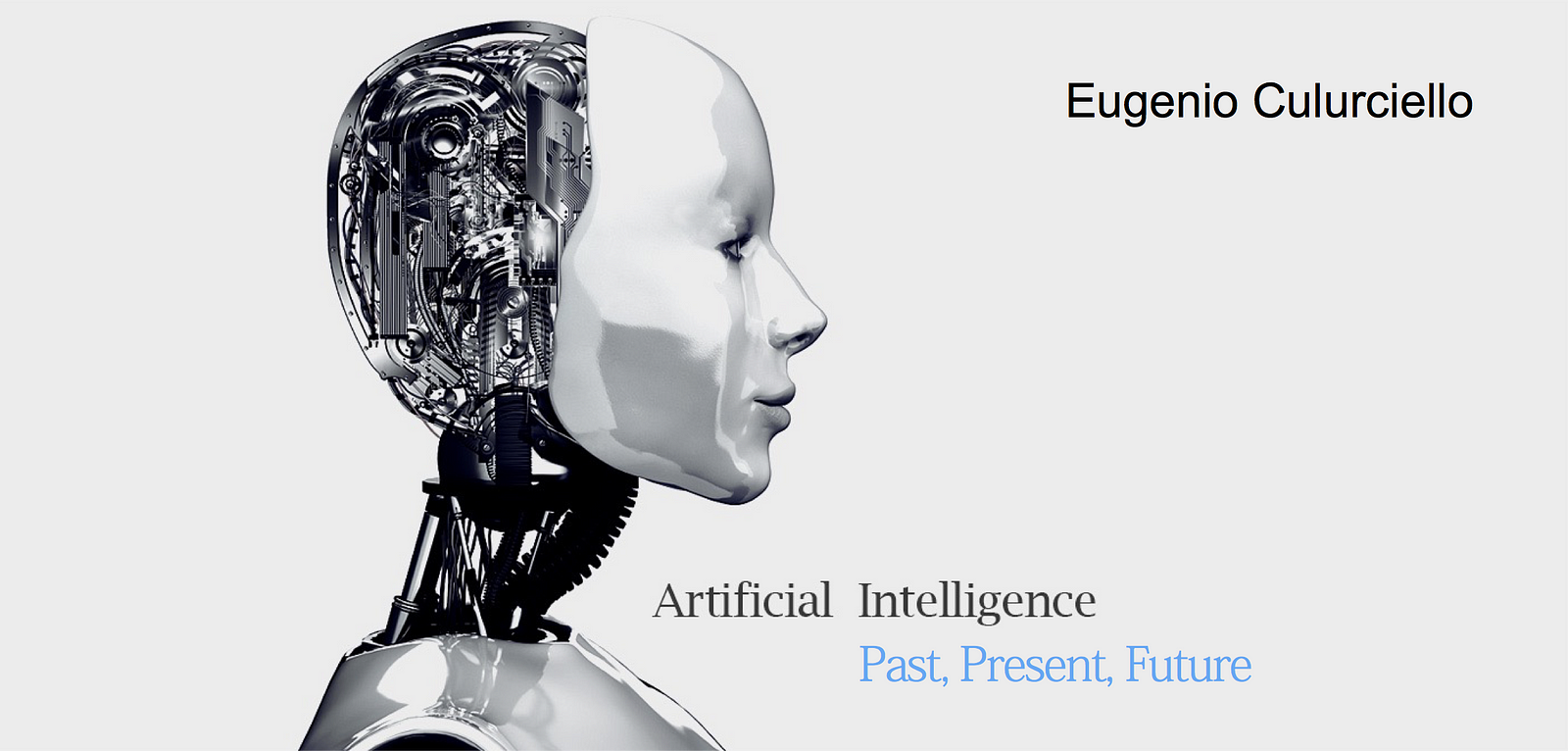
Artificial Intelligence, AI in 2018 and beyond
Or how machine learning is evolving into AI
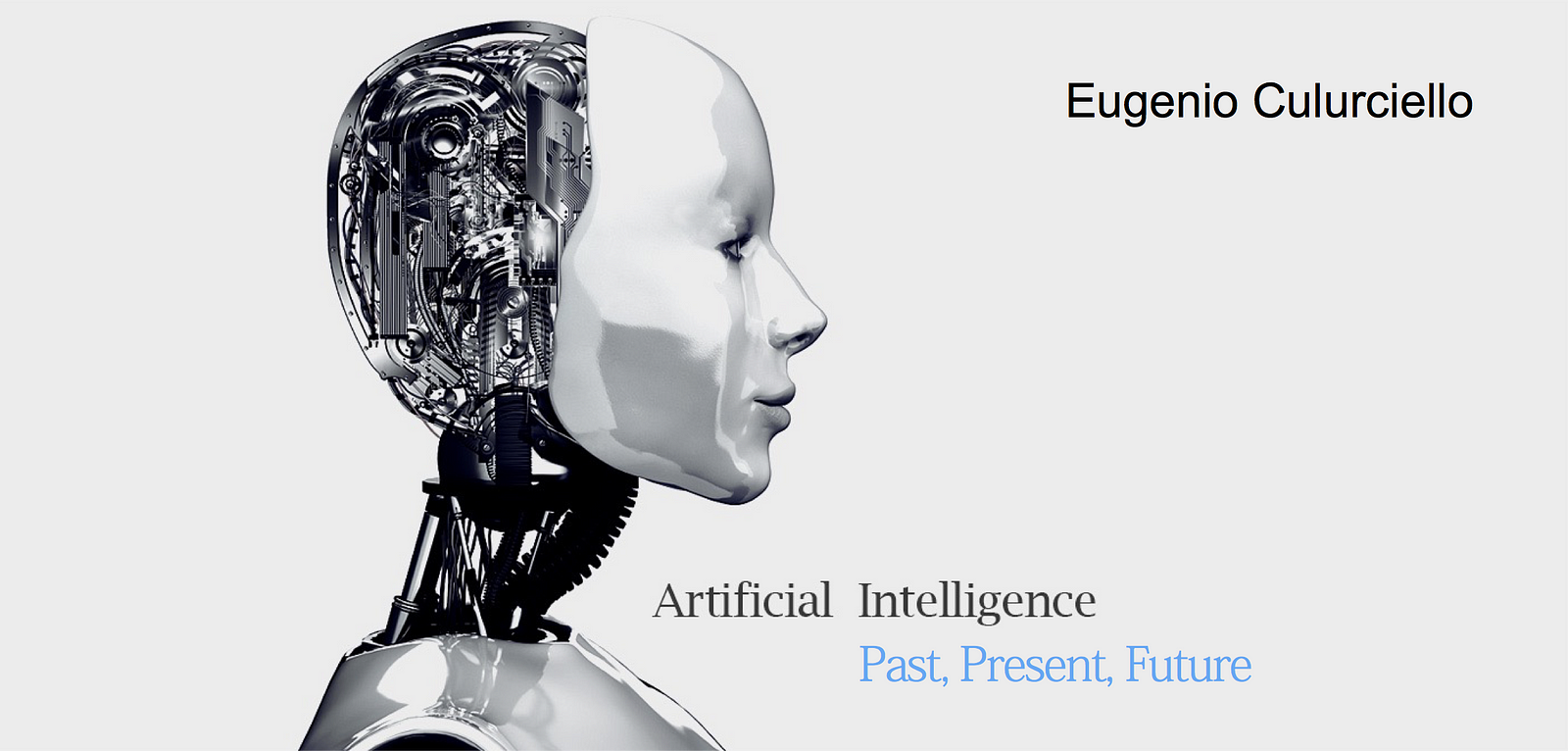
These
are my opinions on where deep neural network and machine learning is
headed in the larger field of artificial intelligence, and how we can
get more and more sophisticated machines that can help us in our daily
routines.
Please
note that these are not predictions of forecasts, but more a detailed
analysis of the trajectory of the fields, the trends and the technical
needs we have to achieve useful artificial intelligence.
Not
all machine learning is targeting artificial intelligences, and there
are low-hanging fruits, which we will examine here also.
Goals
The
goal of the field is to achieve human and super-human abilities in
machines that can help us in every-day lives. Autonomous vehicles, smart
homes, artificial assistants, security cameras are a first target. Home
cooking and cleaning robots are a second target, together with
surveillance drones and robots. Another one is assistants on mobile
devices or always-on assistants. Another is full-time companion
assistants that can hear and see what we experience in our life. One
ultimate goal is a fully autonomous synthetic entity that can behave at
or beyond human level performance in everyday tasks.
Software
Software is defined here as neural networks architectures trained with an optimization algorithm to solve a specific task.
Today
neural networks are the de-facto tool for learning to solve tasks that
involve learning supervised to categorize from a large dataset.
But
this is not artificial intelligence, which requires acting in the real
world often learning without supervision and from experiences never seen
before, often combining previous knowledge in disparate circumstances
to solve the current challenge.
How do we get from the current neural networks to AI?
Neural network architectures
— when the field boomed, a few years back, we often said it had the
advantage to learn the parameters of an algorithms automatically from
data, and as such was superior to hand-crafted features. But we
conveniently forgot to mention one little detail… the neural network
architecture that is at the foundation of training to solve a specific
task is not learned from data! In fact it is still designed by hand.
Hand-crafted from experience, and it is currently one of the major
limitations of the field. There is research in this direction: here and here
(for example), but much more is needed. Neural network architectures
are the fundamental core of learning algorithms. Even if our learning
algorithms are capable of mastering a new task, if the neural network is
not correct, they will not be able to. The problem on learning neural
network architecture from data is that it currently takes too long to
experiment with multiple architectures on a large dataset. One has to
try training multiple architectures from scratch and see which one works
best. Well this is exactly the time-consuming trial-and-error procedure
we are using today! We ought to overcome this limitation and put more
brain-power on this very important issue.
Unsupervised learning
—we cannot always be there for our neural networks, guiding them at
every stop of their lives and every experience. We cannot afford to
correct them at every instance, and provide feedback on their
performance. We have our lives to live! But that is exactly what we do
today with supervised neural networks: we offer help at every instance
to make them perform correctly. Instead humans learn from just a handful
of examples, and can self-correct and learn more complex data in a
continuous fashion. We have talked about unsupervised learning
extensively here.
Predictive neural networks —
A major limitation of current neural networks is that they do not
possess one of the most important features of human brains: their
predictive power. One major theory about how the human brain work is by
constantly making predictions: predictive coding.
If you think about it, we experience it every day. As you lift an
object that you thought was light but turned out heavy. It surprises
you, because as you approached to pick it up, you have predicted how it
was going to affect you and your body, or your environment in overall.
Prediction
allows not only to understand the world, but also to know when we do
not, and when we should learn. In fact we save information about things
we do not know and surprise us, so next time they will not! And
cognitive abilities are clearly linked to our attention mechanism in the
brain: our innate ability to forego of 99.9% of our sensory inputs,
only to focus on the very important data for our survival — where is the
threat and where do we run to to avoid it. Or, in the modern world,
where is my cell-phone as we walk out the door in a rush.
Building
predictive neural networks is at the core of interacting with the real
world, and acting in a complex environment. As such this is the core
network for any work in reinforcement learning. See more below.
We
have talked extensively about the topic of predictive neural networks,
and were one of the pioneering groups to study them and create them. For
more details on predictive neural networks, see here, and here, and here.
Limitations of current neural networks
— We have talked about before on the limitation of neural networks as
they are today. Cannot predict, reason on content, and have temporal
instabilities — we need a new kind of neural networks that you can about read here.
Neural Network Capsules are one approach to solve the limitation of current neural networks. We reviewed them here. We argue here that Capsules have to be extended with a few additional features:
- operation on video frames: this is easy, as all we need to do is to make capsules routing look at multiple data-points in the recent past. This is equivalent to an associative memory on the most recent important data points. Notice these are not the most recent representations of recent frames, but rather they are the top most recent different representations. Different representations with different content can be obtained for example by saving only representations that differ more than a pre-defined value. This important detail allows to save relevant information on the most recent history only, and not a useless series of correlated data-points.
- predictive neural network abilities: this is already part of the dynamic routing, which forces layers to predict the next layer representations. This is a very powerful self-learning technique that in our opinion beats all other kinds of unsupervised representation learning we have developed so far as a community. Capsules need now to be able to predict long-term spatiotemporal relationships, and this is not currently implemented.
Continuous learning
— this is important because neural networks need to continue to learn
new data-points continuously for their life. Current neural networks are
not able to learn new data without being re-trained from scratch at
every instance. Neural networks need to be able to self-assess the need
of new training and the fact that they do know something. This is also
needed to perform in real-life and for reinforcement learning tasks,
where we want to teach machines to do new tasks without forgetting older
ones.
For more detail, see this excellent blog post by Vincenzo Lomonaco.
Transfer learning
— or how do we have these algorithms learn on their own by watching
videos, just like we do when we want to learn how to cook something new?
That is an ability that requires all the components we listed above,
and also is important for reinforcement learning. Now you can really
train your machine to do what you want by just giving an example, the
same way we humans do every!
Reinforcement learning — this
is the holy grail of deep neural network research: teach machines how
to learn to act in an environment, the real world! This requires
self-learning, continuous learning, predictive power, and a lot more we
do not know. There is much work in the field of reinforcement learning,
but to the author it is really only scratching the surface of the
problem, still millions of miles away from it. We already talked about
this here.
Reinforcement
learning is often referred as the “cherry on the cake”, meaning that it
is just minor training on top of a plastic synthetic brain. But how can
we get a “generic” brain that then solve all problems easily? It is a
chicken-in-the-egg problem! Today to solve reinforcement learning
problems, one by one, we use standard neural networks:
- a deep neural network that takes large data inputs, like video or audio and compress it into representations
- a sequence-learning neural network, such as RNN, to learn tasks
Both
these components are obvious solutions to the problem, and currently
are clearly wrong, but that is what everyone uses because they are some
of the available building blocks.
As such results are unimpressive: yes we can learn to play video-games
from scratch, and master fully-observable games like chess and go, but I
do not need to tell you that is nothing compared to solving problems in
a complex world. Imagine an AI that can play Horizon Zero Dawn better than humans… I want to see that!
But this is what we want. Machine that can operate like us.
Our proposal for reinforcement learning work is detailed here. It uses a predictive neural network that can operate continuously and an associative memory to store recent experiences.
No more recurrent neural networks —
recurrent neural network (RNN) have their days counted. RNN are
particularly bad at parallelizing for training and also slow even on
special custom machines, due to their very high memory bandwidth
usage — as such they are memory-bandwidth-bound, rather than
computation-bound, see here for more details. Attention based neural network
are more efficient and faster to train and deploy, and they suffer much
less from scalability in training and deployment. Attention in neural
network has the potential to really revolutionize a lot of
architectures, yet it has not been as recognized as it should. The
combination of associative memories and attention is at the heart of the
next wave of neural network advancements.
Attention has already showed to be able to learn sequences as well as RNNs and at up to 100x less computation! Who can ignore that?
We
recognize that attention based neural network are going to slowly
supplant speech recognition based on RNN, and also find their ways in
reinforcement learning architecture and AI in general.
Localization of information in categorization neural networks — We have talked about how we can localize and detect key-points in images and video extensively here. This is practically a solved problem, that will be embedded in future neural network architectures.
Hardware
Hardware
for deep learning is at the core of progress. Let us now forget that
the rapid expansion of deep learning in 2008–2012 and in the recent
years is mainly due to hardware:
- cheap image sensors in every phone allowed to collect huge datasets — yes helped by social media, but only to a second extent
- GPUs allowed to accelerate the training of deep neural networks
And we have talked about hardware extensively before.
But we need to give you a recent update! Last 1–2 years saw a boom in
the are of machine learning hardware, and in particular on the one
targeting deep neural networks. We have significant experience here, and
we are FWDNXT, the makers of SnowFlake: deep neural network accelerator.
There
are several companies working in this space: NVIDIA (obviously), Intel,
Nervana, Movidius, Bitmain, Cambricon, Cerebras, DeePhi, Google,
Graphcore, Groq, Huawei, ARM, Wave Computing. All are developing custom
high-performance micro-chips that will be able to train and run deep
neural networks.
The
key is to provide the lowest power and the highest measured performance
while computing recent useful neural networks operations, not raw
theoretical operations per seconds — as many claim to do.
But
few people in the field understand how hardware can really change
machine learning, neural networks and AI in general. And few understand
what is important in micro-chips and how to develop them.
Here is our list:
- training or inference? — many companies are creating micro-chips that can provide training of neural networks. This is to gain a portion of the market of NVIDIA, which is the de-facto training hardware to date. But training is a small part of the story and the applications of deep neural networks. For every training step there are a million deployments in actual applications. For example one of the object detection neural network you can now use on the cloud today: it was trained once, and yes on a lot of images, but once trained it can be use by millions of computers on billions of data. What we are trying to say here: training hardware matter as little as the number of times you trained compared to the number of times you use. And making a chipset for training requires extra hardware and extra tricks. This translates into higher power for the same performance, and thus not the best possible for current deployments. Training hardware is important, and a easy modification of inference hardware, but it is not as important as many think.
- Applications — hardware that can provide training faster and at lower power is really important in the field, because it will allow to create and test new models and applications faster. But the real significant step forward will be in hardware for applications, mostly in inference. There are many applications today that are not possible or practical because hardware, and not software, is missing or inefficient. For example our phones can be speech-based assistants, and are currently sub-optimal because they cannot operate always-on. Even our home assistants are tied to the power supplies, and cannot follow us around the house unless we sprinkle multiple microphones or devices around. But maybe the largest application of all is removing the phone screen from our lives, and embedding it into our visual system. Without super-efficient hardware all this and many more applications (small robots) will not be possible.
- winners and losers — in hardware, the winner will be the ones that can operate at the lowest possible power per unit performance, and move into the market quickly. Imagine replacing SoC in cell-phones. Happens every year. Now imagine embedding neural network accelerators into memories. This may conquer much of the market faster and with significant penetration. That is what we call a winner.
About neuromorphic neural networks hardware, please see here.
Applications
We
talked briefly about applications in the Goals section above, but we
really need to go into details here. How is AI and neural network going
to get into our daily life?
Here is our list:
- categorizing images and videos — already here in many cloud services. The next steps are doing the same in smart camera feeds — also here today from many providers. Neural nets hardware will allow to remove the cloud and process more and more data locally: a winner for privacy and saving Internet bandwidth.
- speech-based assistants — they are becoming a part of our lives, as they play music and control basic devices in our “smart” homes. But dialogue is such a basic human activity, we often give it for granted. Small devices you can talk to are a revolution that is happening right now. Speech-based assistants are getting better and better at serving us. But they are still tied to the power grid. The real assistant we want is moving with us. How about our cell-phone? Well again hardware wins here, because it will make that possible. Alexa and Cortana and Siri will be always on and always with you. Your phone will be your smart home — very soon. That is again another victory of the smart phone. But we also want it in our car and as we move around town. We need local processing of voice, and less and less cloud. More privacy and less bandwidth costs. Again hardware will give us all that in 1–2 years.
- the real artificial assistants — voice is great, but what we really want is something that can also see what we see. Analyze our environment as we move around. See an example here and ultimately here. This is the real AI assistant we can fall in love with. And neural network hardware will again grant your wish, as analyzing video feed is very computationally expensive, and currently at the theoretical limits on current silicon hardware. In other words a lot harder to do than speech-based assistants. But it is not impossible, and many smart startups like AiPoly already have all the software for it, but lack powerful hardware for running it on phones. Notice also that replacing the phone screen with a wearable glasses-like device will really make our assistant part of us!
What we want is Her from the movie Her!
- the cooking robot — the next biggest appliances will be a cooking and cleaning robot. Here we may soon have the hardware, but we are clearly lacking the software. We need transfer learning, continuous learning and reinforcement learning. All working like a charm. Because you see: every recipe is different, every cooking ingredient looks different. We cannot hard-code all these options. We really need a synthetic entity that can learn and generalize well to do this. We are far from it, but not as far. Just a handful of years away at the current pace of progress. I sure will work on this, as I have done in the last few years~